
The Edge Multi-Cluster Orchestrator (EMCO) open source project, part of the Linux Foundation Networking umbrella, is a software framework for intent-based deployment of cloud-native applications to a set of Kubernetes clusters, spanning enterprise data centers, multiple cloud service providers and numerous edge locations. It can be leveraged for Private 5G, O-RAN, multi-access edge computing (MEC) applications. EMCO has significant industry momentum from companies like Intel, Equinix, Nokia, and Aarna.ml.
A major benefit of EMCO is extensibility via controllers which perform specific operations. Multiple controllers can be onboarded based on different use cases. Here’s a sample:
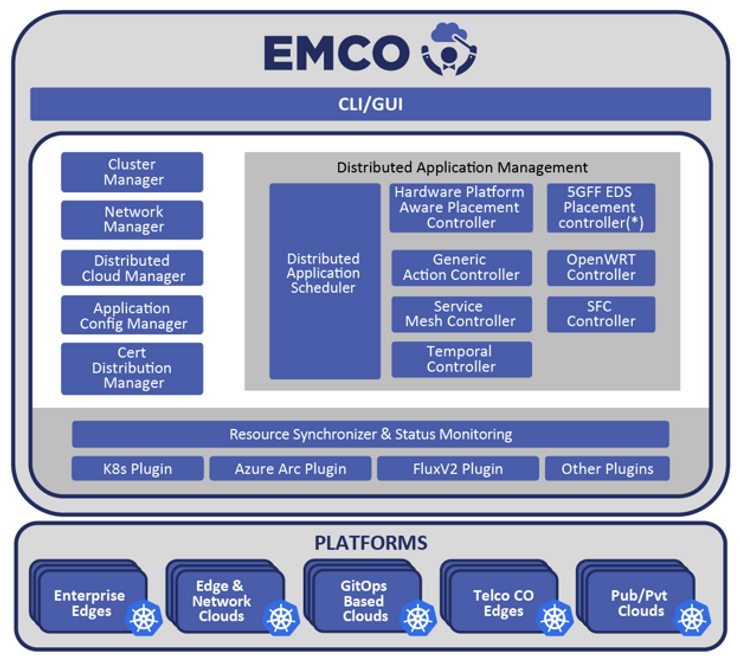
- Cluster Manager - Registers clusters by cluster owners, enables users to onboard target Kubernetes clusters to the platform.
- Network Manager - If secondary interfaces are required for orchestrating the services and applications through EMCO, this controller creates and manages these secondary networks such as exposing existing physical/provider networks into K8s
- Distributed Cloud Manager - Presents a single logical cloud from multiple edges. It is used for stitching the clusters onboarded to the platform.
- Application Config Manager - Enables distribution of application/CNF configuration across Edges & Clouds.
- Cert Distribution Manager - Enrolls CA certificates using tenant specific parent CAs and distributes them across tenant specified K8s clusters.
- Distributed Application Manager - Orchestrates the complex applications (or network services) with the help of various placement controllers. Works with various action controllers to enable secure communication among the microservices.
- Hardware Platform Aware Controller - Enables selection of K8s clusters based on microservices hardware requirements.
- 5GFF EDS Placement Controller - Enables selection of K8s clusters based on latency requirements of application microservices, UE capabilities, 5G Network requirements.
- Generic Action Controller - Allows the customization of K8s resources of applications. Some customization examples include CPU/Memory limits based on destination cluster type.
- Secure Mesh Controller - Auto-configures service mesh (ISTIO/Envoy) of multiple clusters to enable secure L7 connectivity among microservices in various clusters. Also, it can configure ingress/egress proxies to allow external L7 connectivity to/from microservices.
- Secure WAN Controller - Automates fireewall & NAT policies of Cloud native OpenWRT gateways to enable L3/L4 connectivity among microservices and also with external entities.
- Temporal Controller - Allows a way for third parties to develop workflows that need to be executed with complex application (or network service) life cycle.
- SFC Controller - Allows the automation of service function chaining of multiple CNFs. - Resource Synchronizer & Status Monitoring - Manages instantiation of resources to clusters using various plugins to address clusters from various providers.
EMCO 22.03 Highlights
The EMCO 22.03 release brings several improvements, including:
- EMCO GitOps Integration
Resources (services and Kubernetes object) created via EMCO, are now GitOps enabled and deployed in target clusters. These additional controllers push all resources to GitOps in a specific directory structure–enabling any pull request to these resources to be reconciled by agents like Flux V2. This allows for a complete continuous deployment (CD) cycle.
- Modify instantiated logical cloud
There are two kinds of logical clouds – Standard and Admin. Admin logical cloud gives cluster wide authorization to the user. In standard logical cloud user has to specify a name space and resource quotas and permissions about the kind of Kubernetes resources this specific user can access. Until this release, once a logical cloud is created, it could not be modified. In this version, one can modify instantiated logical cloud.
- Enhanced Status Querying/Notifications
In EMCO, a monitoring agent provides the status of resources (e.g. Kubernetes), deployed in the target cluster along with orchestrated applications. The newly added feature is subscription for notifications and enhancements in the status API itself
- New Features Introduced on the Web-Based UI
- RBAC or Role Based Access on the GUI - A way of granting users granular access to Kubernetes based API resources. It implements a security design which provides restricted access to Kubernetes resources based on the role of the user.
- Standard Logical Cloud - Until now, only the admin logical cloud was supported on the GUI, now the standard logical cloud has also been integrated.
- Service Discovery on the GUI - Now integrated is the specific sub-controller, underneath the Distributed Traffic Controller, called the Istio traffic subcontroller. When orchestrating applications across multiple clusters, service discovery helps applications in one cluster to reach out to others.. EMCO creates all the Istio resources to make service discovery possible when deploying the applications across multiple clusters.
- Temporal Workflow Engine
This is a new controller added in EMCO that orchestrates and manages temporal workflows based on use cases.
UI Enhancements: The EMCO 22.03 demo seen here presents a subset of the features of EMCO 22.03 and focussed on the enhancements in the UI. Here you can see how to log in as an admin, onboard the controller, and create a user along with a tenant. EMCO is shown orchestrating two apps -- client and server – across two Kubernetes clusters.
Want to learn more about EMCO? We encourage you to explore the EMCO Wiki, EMCO Repos on GitLab, join the EMCO Mailing list, and attend calls. Send any project related questions to Louis Illuzzi: lilluzzi [at] linuxfoundation [dot] org.